Table of Contents
Imagine a world where doctors can predict diseases before symptoms appear, where personalized treatment plans are developed with precision, and where medical errors are reduced to almost zero. Welcome to the era of AI and Machine Learning are revolutionizing the healthcare industry at an unprecedented pace.
Since their introduction in the 1970s, AI and Machine Learning these cutting-edge technologies have evolved significantly, generating notable advances and transforming the way healthcare is delivered. Today, AI and Machine Learning play a critical role in reducing costs, improving patient outcomes, and increasing overall productivity across the healthcare spectrum.
According to recent surveys, more than 40% of healthcare partners regularly integrate AI and Machine Learning into their operations, harnessing their potential to analyze large amounts of patient data and develop more accurate and personalized treatment plans.
The financial impact is equally striking, as the healthcare AI and Machine Learning market is valued at billions of dollars and is poised for rapid expansion. These technologies not only improve efficiency; They are fundamentally changing the healthcare landscape, moving us closer to a future where healthcare is more accurate, efficient, and accessible.
In this blog, we will discuss the most important innovations and advances in AI and Machine Learning, exploring how they are reshaping the sector and what the future holds. From predictive analytics to personalized medicine, join us to discover the transformative power of Artificial intelligence (AI) and machine learning (ML) in modern healthcare.
Brief Overview of AI and ML Technologies
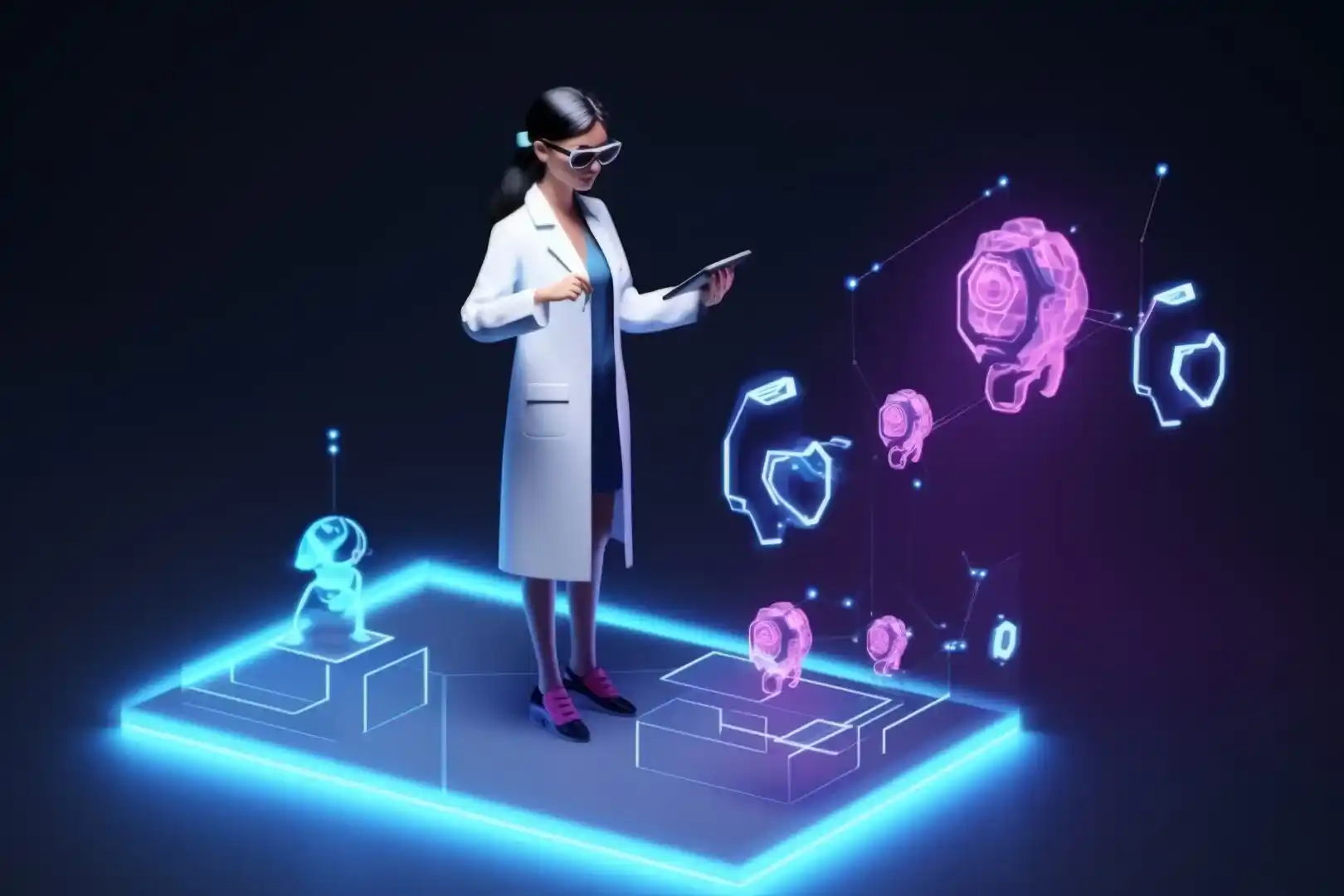
As technology advances, so does digital innovation in healthcare. AI and Machine Learning present exciting potential for medical research to improve diagnostic accuracy, personalized care, and the discovery of creative solutions.
Data and Information Analysis:
AI and Machine Learning can process large amounts of data to uncover vital information. The use of predictive algorithms validates doctors’ thinking and judgments. In this situation, AI and Machine Learning can confirm the treatment plan by identifying a patient with a comparable medical history who received the same treatment.
Case Study: IBM Watson for Oncology
IBM Watson for Oncology uses AI and Machine Learning to analyze large volumes of medical literature and patient data to aid in the diagnosis and treatment of cancer. In a pilot study conducted in India, Watson for Oncology achieved a 93% accuracy rate in recommending treatment options, compared to 96% for expert oncologists. This demonstrates the ability of AI and Machine Learning to manage large amounts of data and facilitate decision-making.
Diagnosing and Treating Diseases:
From chronic diseases like cancer to X-rays, AI and Machine Learning are being used to develop effective and accurate technologies to help treat people suffering from these diseases. AI has significant advantages over conventional methods of clinical analysis and decision-making.
Case Study: Google Health AI for Retinal Diseases
Google Health’s artificial intelligence system for detecting retinal diseases has shown promising results. In a study of 3,000 patients, the AI and Machine Learning system achieved a diagnostic accuracy of 94.6% in detecting diabetic retinopathy and macular edema, compared to 87.2% for human experts. This highlights the potential of AI and Machine Learning to improve diagnostic accuracy.
Clinical Decision Support:
AI and Machine Learning algorithms improve system accuracy by understanding training data, allowing users to gain unprecedented insights into care procedures, diagnoses, and patient outcomes.
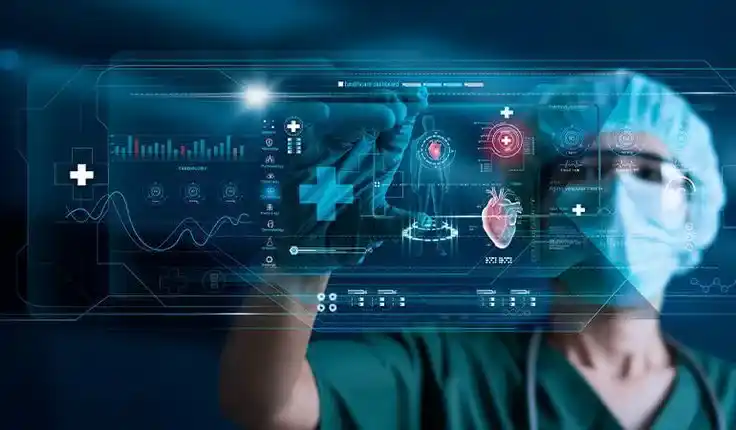
Hyscaler’s Use of AI and ML
Hyscaler leverages cutting-edge AI and Machine Learning technologies to drive innovation and improve outcomes across diverse industries, including healthcare. Its approach includes:
Predictive Analytics:
Hyscaler uses machine learning algorithms to analyze large data sets, allowing healthcare providers to predict patient outcomes, personalize treatment plans, and improve diagnostic accuracy.
Case Study: Predictive Analytics in Chronic Disease Management
A healthcare provider using Hyscaler’s predictive analytics platform was able to reduce hospital readmissions for patients with chronic conditions by 20% through early intervention and model-based personalized care plans.
AI-Enhanced Diagnosis:
By integrating AI, Hyscaler is developing tools that aid early and accurate detection of diseases, particularly in areas such as oncology and radiology. These tools help healthcare professionals identify conditions more quickly and accurately than traditional methods.
Case Study: Hyscaler’s AI Tool for Oncology
Hyscaler’s AI tool for oncology was tested in a clinical trial and achieved a 90% accuracy rate in detecting early-stage cancer, compared to 80% for conventional methods, demonstrating the effectiveness of AI in improving diagnostic capabilities.
Personalized Care Solutions:
Hyscaler uses AI to create personalized care plans based on patient data. This personalization ensures that each patient receives the most effective treatment, improving overall health outcomes.
Case Study: Personalized Diabetes Management
Hyscaler’s AI-powered personalized care solutions helped improve blood sugar control in diabetes patients by 25% by tailoring treatment plans based on individual patient data and mode factors of life.
Operational Efficiency:
ai-powered mobile app features and intelligent systems implemented by Hyscaler streamline healthcare operations, reduce administrative burdens, and enable healthcare professionals to focus more on patient care.
Through its AI and Machine Learning development services, Hyscaler aims to revolutionize healthcare by providing advanced technological solutions that improve diagnostic accuracy, treatment efficiency and quality of patient care.
According to Acumen Research, the market for AI in health care is estimated to reach $8 billion by 2026.
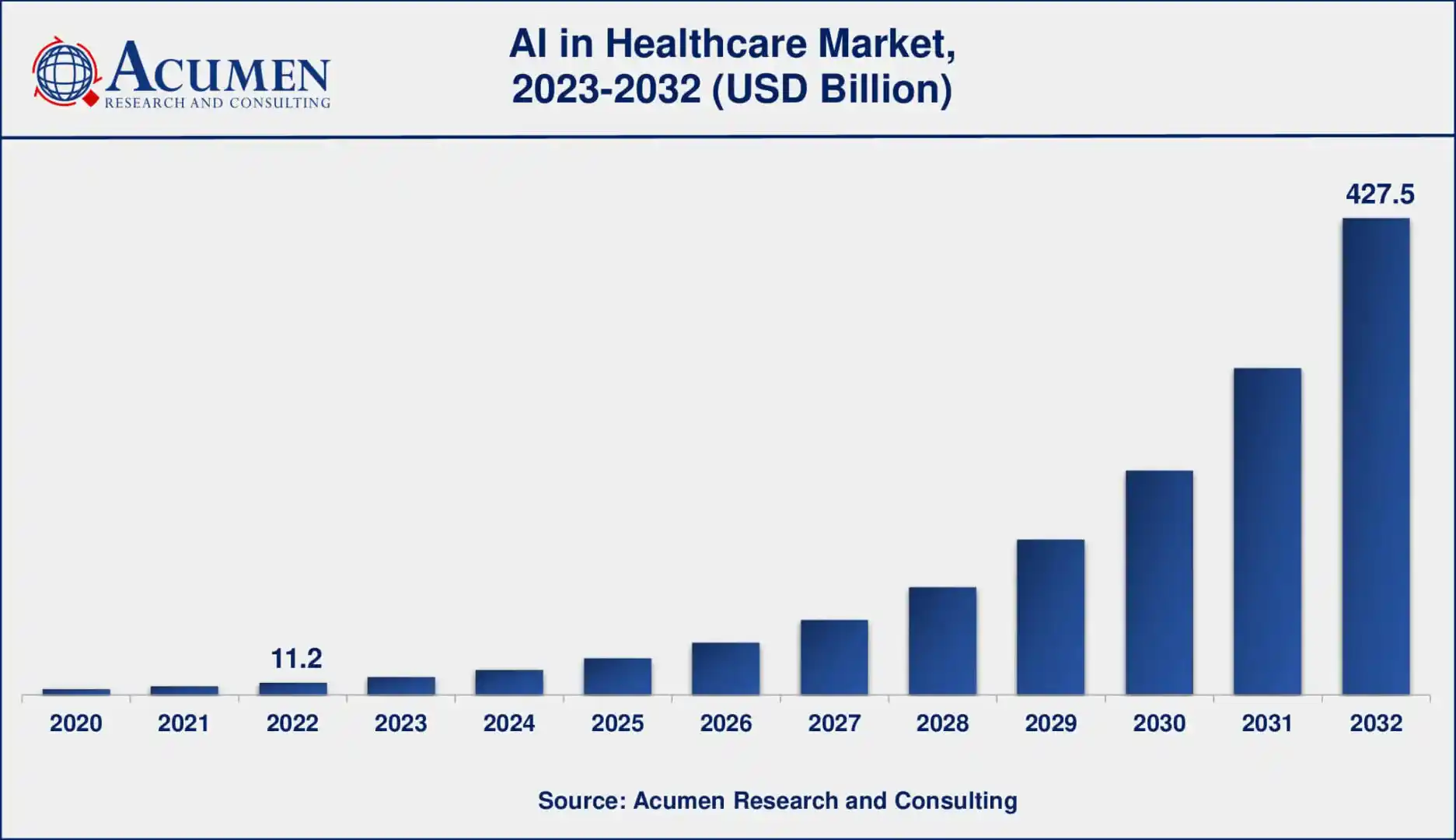
Benefits of AI and ML in Healthcare
We are all witnessing the widespread use of AI and machine learning in the medical sector, and here are some other examples.
1. Improve Accuracy
AI determines the vast amount of medical data required to accurately diagnose a disease at an early stage. It helps minimize diagnostic errors and allows medical experts to process much information.
2. Increase response times
AI and ML also help deliver test results in a shorter time than the manual diagnosis method. AI not only helps reduce costs but also facilitates timely processing.
3. Predictive Analysis and Clinical Advice
AI and ML are used to predict potential errors based on genetic conditions, patient lifestyle, medical records, etc.
AI and ML analyze patients’ electronic medical records in real-time and also predict treatment outcomes. Health professionals also exploit the potential of A&ML to evaluate the favorable treatment of individuals.
4. Personalized medications
AI-powered mobile app features can help patients receive personalized therapies and medication regimens. This is accomplished by conducting a thorough review of patients’ underlying genetic history, clinical data, and medical outcomes to detect patterns and relationships.
During the clinical study phase, AI can be used to create personalized medications for certain medical conditions.
5. Data visualization
ML can be used to create three-dimensional representations of biological data, including RNA sequences, protein structures, and genetic profiles. It helps surgeons perform complex tasks throughout the surgical procedure, providing a clearer picture of the region in which they operate and demonstrating how to perform operations.
Challenges and Solutions in Implementing AI and ML
1. Data Security
One of the most serious problems with the use of AI in healthcare is unauthorized access to patient or consumer data. Information in medical records is considered confidential information and requires additional safeguards.
Artificial intelligence (AI) and machine learning (ML) technologies work best with large data sets, but the lack of information makes it difficult to develop effective methods for accurate diagnosis.
2. Uncertainties About AI
AI has had a huge impact on the medical field by recognizing diseases at an early stage. This has raised concerns about the use of AI in routine care operations.
3. Training Materials for AI and ML Integration
Another major barrier to AI adoption in healthcare is the lack of technical training for stakeholders. Healthcare personnel must understand how artificial intelligence technology is applied in various care units. However, AI technology could be difficult to understand. It is difficult to provide personalized training to different resources.
4. Lack of an Execution Framework
While there are a large number of studies on how AI can change the medical field, there are few studies on how to implement AI in healthcare. This results in minimal awareness on the part of health officials, professionals, and patients.
Many people are unaware of how AI can be integrated into current healthcare procedures and systems. To solve this problem, healthcare professionals must have a robust framework to effectively integrate AI into healthcare.
5. Ethical Issues
Accountability is extremely important in the medical industry. We have little understanding of how artificial intelligence produces results as it trains itself based on the input data provided. It can be difficult to determine liability if an inaccurate diagnosis leads to an unpleasant outcome.
Furthermore, the lack of a common ethical framework or guidance for the implementation of AI for medical purposes makes it difficult to establish accountability.
Conclusion
Artificial intelligence (AI) and machine learning (ML) technologies are transforming the healthcare industry and offer numerous benefits, such as improved diagnostic accuracy, faster response times, predictive analytics, personalized medications, and improved data visualization. However, there are challenges in implementing these technologies, including data security, uncertainties regarding AI, lack of technical training, lack of an implementation framework, and ethical issues. Addressing these challenges is crucial to maximizing the potential of AI applications and machine learning in healthcare.
As the healthcare AI and ML market continues to grow, organizations like Hyscaler are leading the way in developing and implementing innovative solutions that improve patient care and operational efficiency. By adopting these technologies and overcoming the challenges associated with them, the healthcare industry can move toward a future where healthcare is more accurate, efficient, and accessible.