Table of Contents
In today’s digital landscape, fraud detection has become more critical than ever, with both individuals and businesses facing increasing risks of financial fraud. Traditional methods often fail to keep pace with evolving fraud tactics, leading many organizations to adopt AI-driven solutions for better accuracy and efficiency.
In this article, we’ll explore how AI fraud detection works, and its applications across various sectors, and introduce seven powerful tools that can enhance financial security.
What is AI Fraud Detection?
AI fraud detection leverages artificial intelligence and machine learning (ML) algorithms to identify and prevent fraudulent activities. Unlike traditional rule-based systems, AI fraud detection solutions can learn from vast datasets, adapt to new fraud patterns, and make real-time decisions. By analyzing transaction patterns, behaviors, and other data points, AI can flag anomalies and help organizations mitigate risks swiftly.
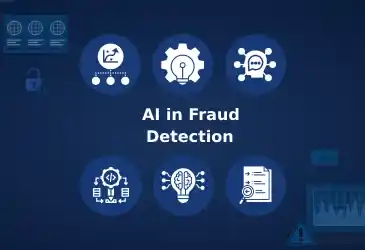
How Does AI Fraud Detection Work?
AI fraud detection systems typically follow these key steps:
- Data Collection: These systems gather data from various sources, including transaction records, customer profiles, and social media activity, to create a comprehensive understanding of normal behavior.
- Pattern Recognition: Machine learning algorithms analyze historical and real-time data to identify normal patterns. The AI system then flags any deviations from these patterns as potential fraud.
- Anomaly Detection: Using sophisticated algorithms like neural networks or decision trees, the system detects anomalies—such as unusual transaction sizes or locations—signaling potential fraud.
- Risk Scoring: Each transaction is evaluated and given a risk score that reflects the probability of potential fraud. Transactions with a risk score exceeding a specific threshold are marked for additional review.
- Continuous Learning: The AI models learn from previous fraud cases, improving accuracy and minimizing false positives over time.
By automating these steps, AI fraud detection systems save time and reduce human error, making fraud detection more effective and efficient.
AI Fraud Detection Use Cases in Different Sectors
AI fraud detection can be applied across various industries to improve security and efficiency. Here are a few examples:
- Banking and Financial Services: AI fraud detection is widely used to analyze transaction patterns and detect suspicious behavior in real time. It helps prevent credit card fraud, money laundering, and identity theft.
- E-commerce: For online retailers, AI helps detect fraudulent orders, account takeovers, and phishing attacks, protecting both the business and its customers.
- Insurance: AI fraud detection in insurance assesses claims for fraud risk by analyzing historical data, customer behaviors, and unusual patterns.
- Healthcare: In healthcare, AI identifies potential fraud in billing, prescriptions, and patient data by analyzing records for inconsistencies.
- Telecommunications: AI helps telecom companies spot fraud in areas like subscription fraud, fake accounts, and phone scams, minimizing revenue loss.
7 Tools for Secure Finances
Here’s a look at seven powerful AI fraud detection tools that help organizations secure their finances:
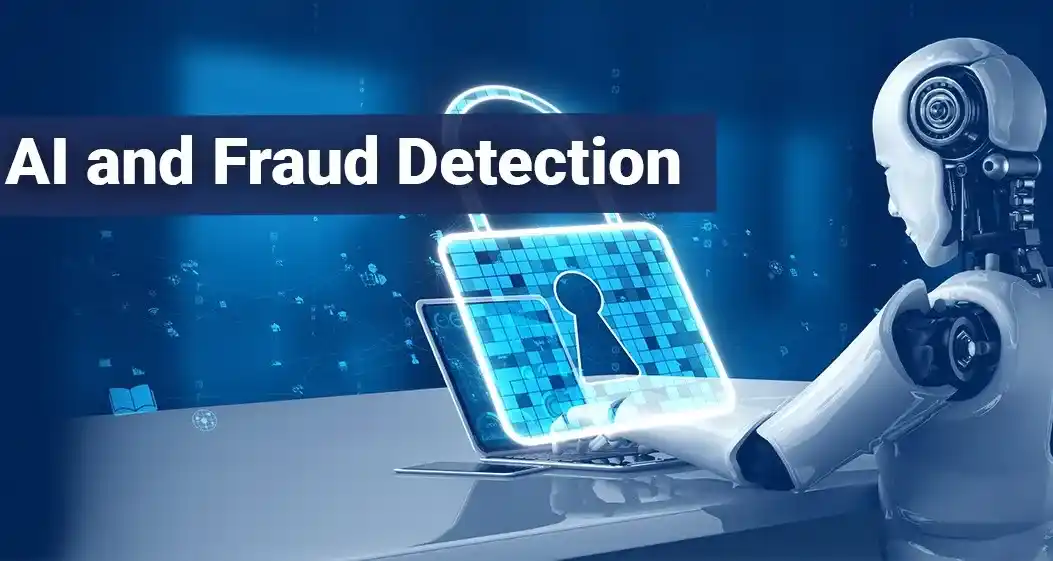
- Fraud.netFraud.net offers a comprehensive fraud detection solution using AI and big data analytics. Designed for e-commerce, banking, and travel sectors, it analyzes user behavior, transaction history, and IP data to detect suspicious activities. Its customizable dashboards allow teams to visualize and act on fraud insights quickly.
- SAS Fraud ManagementSAS provides an enterprise-grade fraud management system that uses AI to monitor and prevent fraud in real time. Known for its advanced analytics, SAS can detect unusual behavior in transactions, account activity, and more, making it ideal for financial institutions.
- KountKount uses machine learning to detect and prevent fraud in digital payments, e-commerce, and finance. It tracks more than 13 billion transactions annually, building unique customer identity profiles to prevent account takeovers and other fraud types.
- DarktraceDarktrace offers an AI-driven cybersecurity solution that adapts to new threats, including financial fraud. Its system uses machine learning to analyze network behavior and identify potential breaches. Darktrace’s “immune system” technology mimics human immune responses, making it highly adaptable to emerging fraud patterns.
- IBM TrusteerIBM Trusteer is a fraud prevention platform that offers AI-based solutions for online banking security. Trusteer can identify high-risk transactions, detect malware, and prevent account takeover attempts, making it a popular choice among banks and financial institutions.
- DataVisorDataVisor combines machine learning with big data to detect sophisticated fraud attacks. It uses unsupervised machine learning, which doesn’t require labeled data, making it effective in identifying new fraud patterns. DataVisor is widely used in banking, e-commerce, and social platforms.
- ZensedZensed is an AI-powered tool designed to detect fraud in e-commerce and financial transactions. Its predictive models analyze customer data to assess risk and prevent fraud in real time. Zensed’s models continuously update to adapt to new threats, providing businesses with up-to-date protection.
Conclusion
AI fraud detection has revolutionized how companies manage financial risks, providing faster, more accurate results than traditional methods. With tools like Fraud.net, SAS, Kount, Darktrace, IBM Trusteer, DataVisor, and Zensed, businesses across sectors can better protect their finances against evolving fraud tactics.
Adopting these AI-driven solutions empowers companies to safeguard their assets, build customer trust, and stay ahead in the fight against financial fraud.