Table of Contents
DeepSeek AI, a Chinese AI startup, has announced the launch of the DeepSeek LLM family, a set of open-source large language models (LLMs) that achieve remarkable results in various language tasks. The DeepSeek LLM family consists of four models: DeepSeek LLM 7B Base, DeepSeek LLM 67B Base, DeepSeek LLM 7B Chat, and DeepSeek 67B Chat. These models represent a significant advancement in language understanding and application.
A Breakthrough in Language Comprehension
One of the main features that distinguishes the DeepSeek LLM family from other LLMs is the superior performance of the 67B Base model, which outperforms the Llama2 70B Base model in several domains, such as reasoning, coding, mathematics, and Chinese comprehension. The 67B Base model demonstrates a qualitative leap in the capabilities of DeepSeek LLMs, showing their proficiency across a wide range of applications.
Another notable achievement of the DeepSeek LLM family is the LLM 7B Chat and 67B Chat models, which are specialized for conversational tasks. The LLM 67B Chat model achieved an impressive 73.78% pass rate on the HumanEval coding benchmark, surpassing models of similar size. It also scored 84.1% on the GSM8K mathematics dataset without fine-tuning, exhibiting remarkable prowess in solving mathematical problems.
DeepSeek LLM: An Open-Source Resource for AI Research and Application
DeepSeek AI has decided to open-source both the 7 billion and 67 billion parameter versions of its models, including the base and chat variants, to foster widespread AI research and commercial applications. The models are available on GitHub and Hugging Face, along with the code and data used for training and evaluation.
To ensure unbiased and thorough performance assessments, DeepSeek AI designed new problem sets, such as the Hungarian National High-School Exam and Google’s instruction following the evaluation dataset. These evaluations effectively highlighted the model’s exceptional capabilities in handling previously unseen exams and tasks. The problem sets are also open-sourced for further research and comparison.
A Result of Meticulous Data Collection and Training Process
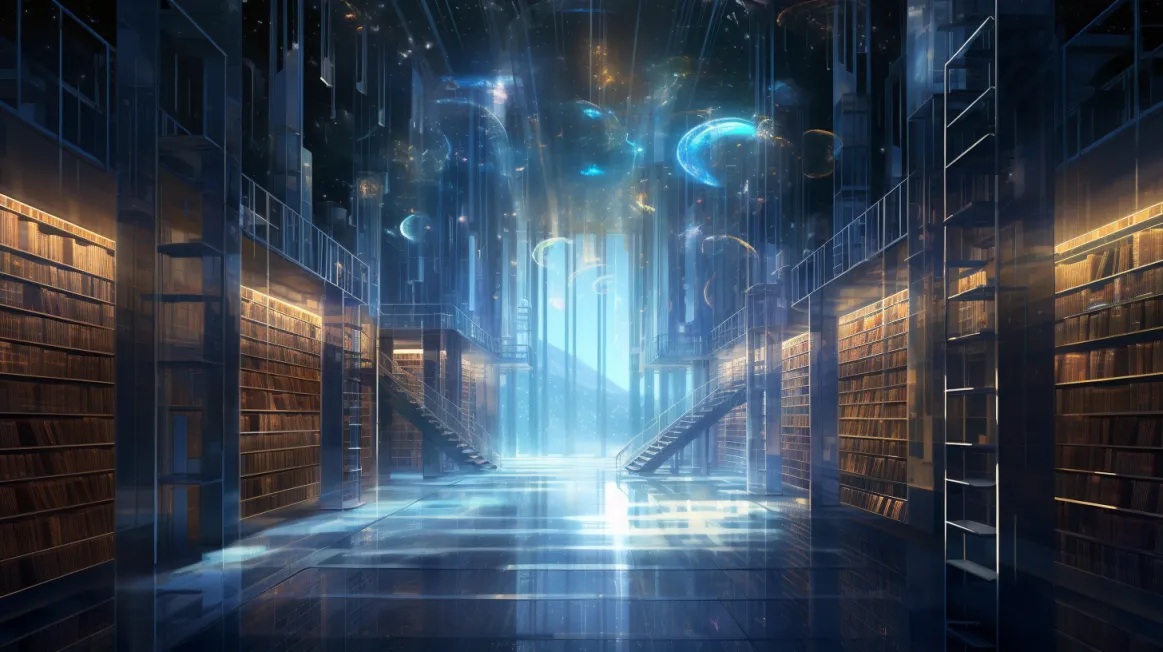
The startup provided insights into its meticulous data collection and training process, which focused on enhancing diversity and originality while respecting intellectual property rights. The multi-step pipeline involved curating quality text, mathematical formulations, code, literary works, and various data types, implementing filters to eliminate toxicity and duplicate content.
DeepSeek’s language models, designed with architectures akin to LLaMA, underwent rigorous pre-training. The 7B model utilized Multi-Head attention, while the 67B model leveraged Grouped-Query Attention. The training regimen employed large batch sizes and a multi-step learning rate schedule, ensuring robust and efficient learning capabilities.
By spearheading the release of these state-of-the-art open-source LLMs, DeepSeek AI has marked a pivotal milestone in language understanding and AI accessibility, fostering innovation and broader applications in the field.
What is the difference between DeepSeek LLM and other language models?
DeepSeek differs from other language models in that it is a collection of open-source large language models that excel at language comprehension and versatile application. In key areas such as reasoning, coding, mathematics, and Chinese comprehension, LLM outperforms other language models.
It also demonstrates exceptional abilities in dealing with previously unseen exams and tasks. The LLM was trained on a large dataset of 2 trillion tokens in both English and Chinese, employing architectures such as LLaMA and Grouped-Query Attention. By open-sourcing its models, code, and data, DeepSeek LLM hopes to promote widespread AI research and commercial applications.
Other language models, such as Llama2, GPT-3.5, and diffusion models, differ in some ways, such as working with image data, being smaller in size, or employing different training methods.