Table of Contents
Google has introduced a new foundation of artificial intelligence (AI) models fine-tuned for the healthcare industry, called MedLM. MedLM is currently available in the US and is based on Google’s previous research in healthcare and medicine, Med-PaLM 2, a large language model trained on medical data.
MedLM consists of two models with separate endpoints, giving customers more flexibility for their use cases. MedLM can perform tasks such as answering medical questions and summarizing medical information.
MedLM: A versatile and powerful AI model for healthcare
The first model in MedLM is a larger variant that can handle complex and intricate tasks, such as generating medical reports or analyzing clinical data.
The second model is a medium-sized variant that can be easily fine-tuned and scaled for various applications, such as creating medical chatbots or extracting medical entities.
Both models are informed by specific healthcare and life sciences requirements and are designed to augment AI adoption in healthcare, from basic functions to sophisticated workflows.
A Collaborative and Innovative AI Model for Healthcare
Google has partnered with several organizations, such as HCA Healthcare, BenchSci, Accenture, and Deloitte, to apply MedLM for different stages of healthcare, improving performance and efficiency in existing projects.
For example, It is used by HCA Healthcare in collaboration with Augmedix, a platform that uses natural language processing to convert clinician-patient conversations into draft medical notes, complying with healthcare regulations. This automation not only enhances performance but also contributes to time savings, reduced burnout, and improved patient care.
Another example is BenchSci, a company that uses MedLM within its ASCEND platform, an AI-powered evidence engine that aims to accelerate drug discovery by improving the speed and quality of pre-clinical research.
ASCEND uses it to boost the identification and classification of biomarkers, which are indicators of biological processes or diseases, thereby streamlining the scientific discovery process.
Google also works with Accenture to leverage generative AI, a type of AI that can create new content or data, to improve patient access, experience, and outcomes. By integrating Google Cloud’s Claims Acceleration Suite and MedLM, healthcare organizations can discover new insights, leading to better patient outcomes.
Additionally, Google and Deloitte are piloting MedLM’s capabilities to simplify information retrieval from provider directories and benefits documents, helping enterprise contact centers agents find suitable providers based on various criteria.
A Promising and Responsible AI Model for Healthcare
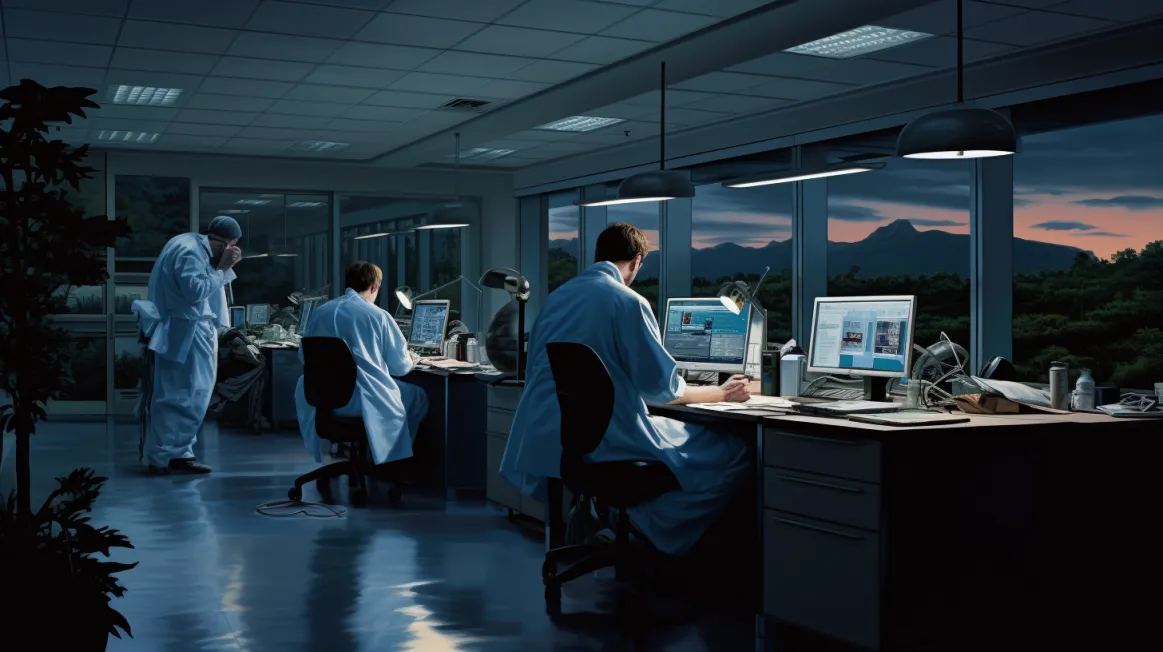
All these projects demonstrate how MedLM can help the growth of AI in the healthcare and medicine industry. Looking ahead, Google plans to expand the MedLM suite by adding Gemini-based models, which are models that can generate natural language and code, to offer even more capabilities in the coming months. The collaborative efforts with industry leaders highlight the transformative potential of generative AI in healthcare.
As technology advances, Google remains committed to ensuring a safe and responsible use of AI in healthcare, working closely with practitioners, researchers, and healthcare organizations to push forward breakthrough research in health and life sciences.
How does natural language processing work in healthcare?
Natural language processing (NLP) is a branch of AI that deals with the interpretation and manipulation of human-generated spoken or written data. NLP can assist healthcare organizations in maximizing the value of unstructured data such as clinical notes, lab reports, literature, and social media by converting it into structured data that can be analyzed and used for a variety of purposes.
By leveraging the potential of unstructured data, NLP is a powerful and versatile technology that can improve healthcare delivery, research, and innovation. However, there are some challenges and limitations to NLP, such as data quality, privacy, security, ethics, and regulation, that must be carefully considered and used responsibly.